Session #1: 5th Oct, 7 pm ET.
Why this matters
We are coming together to participate in a Kaggle Competition, where we will build a model that infers gene to protein expression profiles in cells at various stages of development. This will help biomedical scientists and doctors providing services to patients with cell development issues – particularly doctors who are oncologists (who study cancer) or biologists who are looking to study cell development.
Pre-Requisites - Some familiarity with biological concepts on the molecular level, specifically around the “central dogma” of biology, but nothing else. Familiarity with research methods around cell characteristic profiling is encouraged, but not necessary.
Â
Â
Leads / Advisors
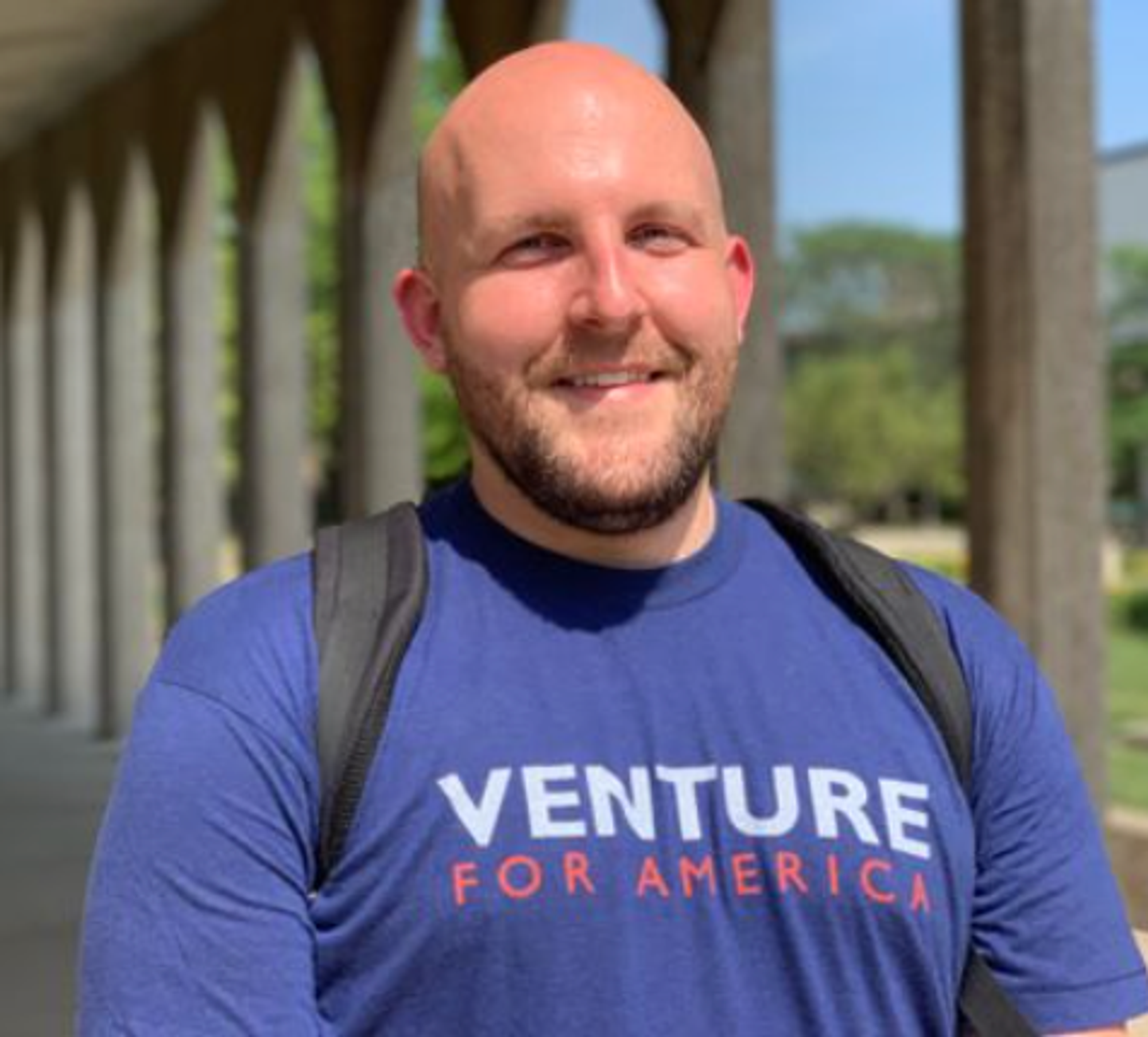
~ Kevin McPherson | Co-lead
Machine Learning Scientist at Knowledge Futures Group
âť—Â Weekly Meeting Time: 7-8 PM ET on Wednesdays
âť—Â Slack channel: click here to join [Reach out to ammar@ai.science if issues]
Â
Â
Context
TL;DR
You will develop a model trained on a subset of 300,000-cell time course dataset of CD34+ hematopoietic stem and progenitor cells (HSPC) from four human donors at five time points generated for this competition by Cellarity, a cell-centric drug creation company.
Full → Kaggle’s Website.
Â
Â
Minimum Success
Minimum Goal:
- A model to submit for Kaggle’s evaluation
- Comparable accuracy to models previously published in the literature.
- Take Kaggle Bronze 🥉
Stretch Goal:
- Take Kaggle Gold 🥇 !
- Win the $25K prize purse!
- Visualize the profiles of cells in an Internet-facing application
- Develop an MVP for doctors and researchers to use to better understand cellular development
Â
Â
Early Audience Hypothesis
The target audience includes researchers in the biomedical sciences and doctors providing services to patients with cell development issues – particularly doctors who are oncologists (who study cancer) or biologists who are looking to study cell development. In both cases, this could be served e.g. as an app, with the goal to be used as a tool in diagnostic settings and as a mirror for understanding cellular development.
Â
Â
Starting Dataset & Description (taken from Kaggle’s website)
The dataset for this competition comprises single-cell multiomics data collected from mobilized peripheral CD34+ hematopoietic stem and progenitor cells (HSPCs) isolated from four healthy human donors. More information about the cells can be found on the vendor website.
Measurements were taken at five time points over a ten-day period. From each plate at each time point, cells were collected for measurement with two single-cell assays. The first is the 10x Chromium Single Cell Multiome ATAC + Gene Expression technology (Multiome) and the second is the 10x Genomics Single Cell Gene Expression with Feature Barcoding technology using the TotalSeq™-B Human Universal Cocktail, V1.0 (CITEseq).
If you've never worked with this data type before, we've included some links at the bottom of this description.
Each assay technology measures two modalities. The Multiome kit measures chromatin accessibility (DNA) and gene expression (RNA), while the CITEseq kit measures gene expression (RNA) and surface protein levels.
Following the central dogma of molecular biology:Â DNA --> RNA-->Protein, your task is as follows:
- For the Multiome samples: given chromatin accessibility, predict gene expression.
- For the CITEseq samples: given gene expression, predict protein levels.
Learning Resources
- Open Problems - About multimodal single-cell data - An info page created last year to explain multimodal data to those new to the data type.
- Models Inferences and Algorithms - Uniting to compete on multimodal single-cell analysis - A talk presented by the competition organizers at the Broad Institutes Models, Inferences, and Algorithms seminar provides background and motivation for the competition.
Â
Â
Â
Onboarding [Technical + Domain Knowledge]
Our tentative solution architecture
Recipes
Tentative Timeline
# | Major Milestones | Expected Finish |
1 | Get to a baseline model | 1 week [~10 hrs / wk] |
2 | We collaborate to innovate | 6 weeks [~ 3hrs / wk] |
4 | Deployment of application | 2 weeks [~ 3hrs / wk] |
5 | Paper writing and submission | 1 weeks [TBA] |
Â
Â
Â
Key Questions to Answer
- What do we have as input? 300,000-cell time course dataset of CD34+ hematopoietic stem and progenitor cells (HSPC)
- What are we trying to do? Predict how DNA, RNA, and protein measurements co-vary in single cells (tasked with predicting a paired modality measured in the same cell).
- Why do we need to do it? How does it help? Accelerates innovation in methods of mapping genetic information across layers of cellular state.
- Dictionary: We need a small explanation of the CD34+ HSPC and how things are normally.
Â
Â
Why join?
Aggregate Intellect hosts one of the most diverse ML communities in the world. Over the course of the working group
- You’ll get an immersion into that community & walk out with some cool new friends.
- Get spotlighted for your efforts on our community website!
- Work on a problem in the bioinformatics sphere that has real-world implications
- Publish your work on arXiv as a way to showcase complex problem-solving skills
- Your work will help accelerate innovation in methods of mapping genetic information across layers of cellular state. If we can predict one modality from another, we may expand our understanding of the rules governing these complex regulatory processes.
Â
Â
Â
Â
Â
Â
Â
Â
Â
Â