Starts: May 23 - 6 pm ET
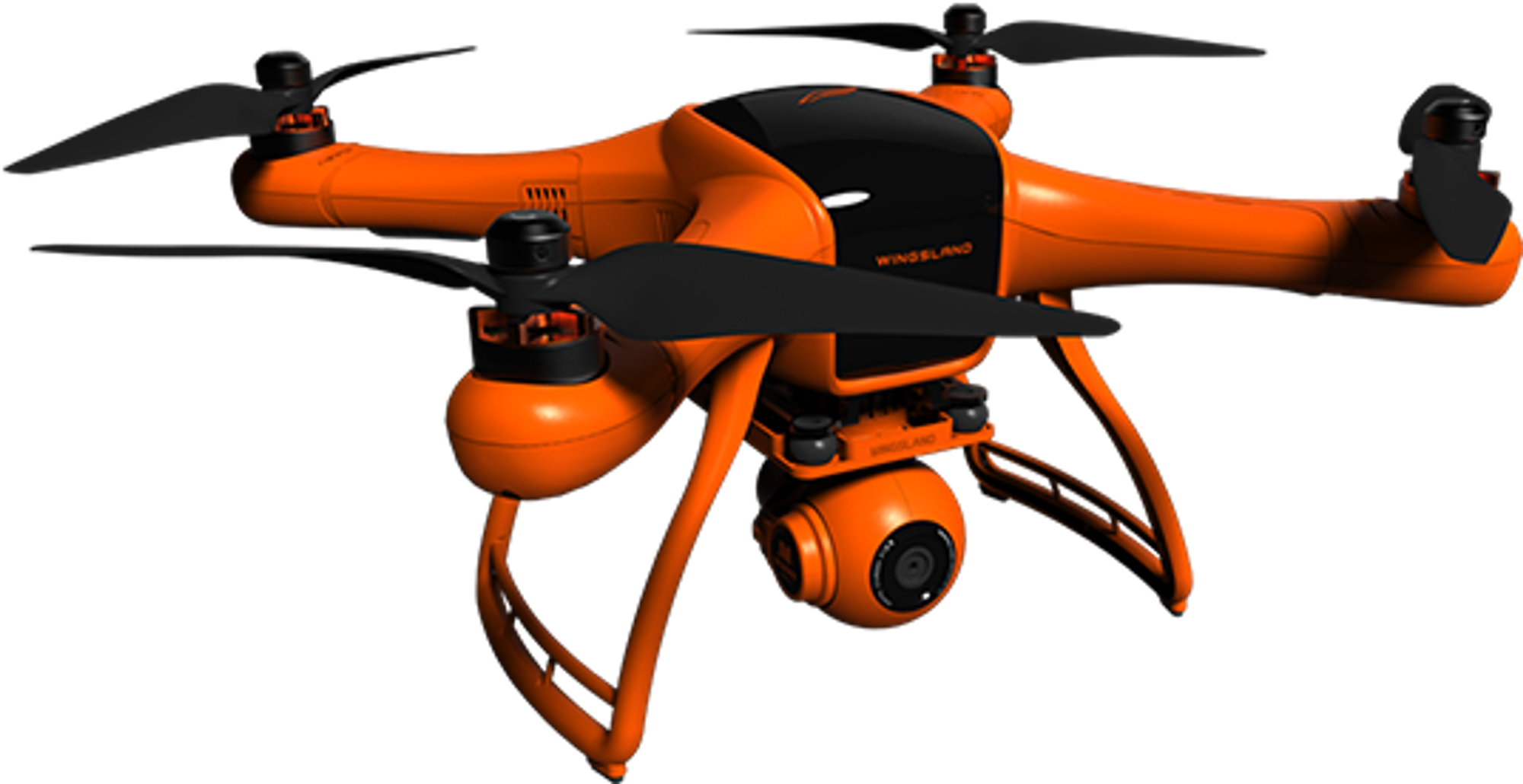
High resolution drone images provide detailed snapshots of the cities’ landscape. In this working group, we examine the capabilities of multiple ML techniques trained on custom-collected data to develop classification algorithms.
Prior experience using Python is required for this project. We recommend that user’s are also familiar with Google Colab, Tensorflow and Numpy for ML-related modelling tasks. No previous remote sensing knowledge is needed for this project. Please watch the videos in the “Other Useful links + Resources” section to get a clear high level understanding of the whole project: [ https://www.notion.so/aggregate-intellect/7e815b3a5f4e48b3ac6d92fc2c19e7cd?v=aaf9ff169e8e4687916fc560031a8734].
Working Group Leads / Advisors
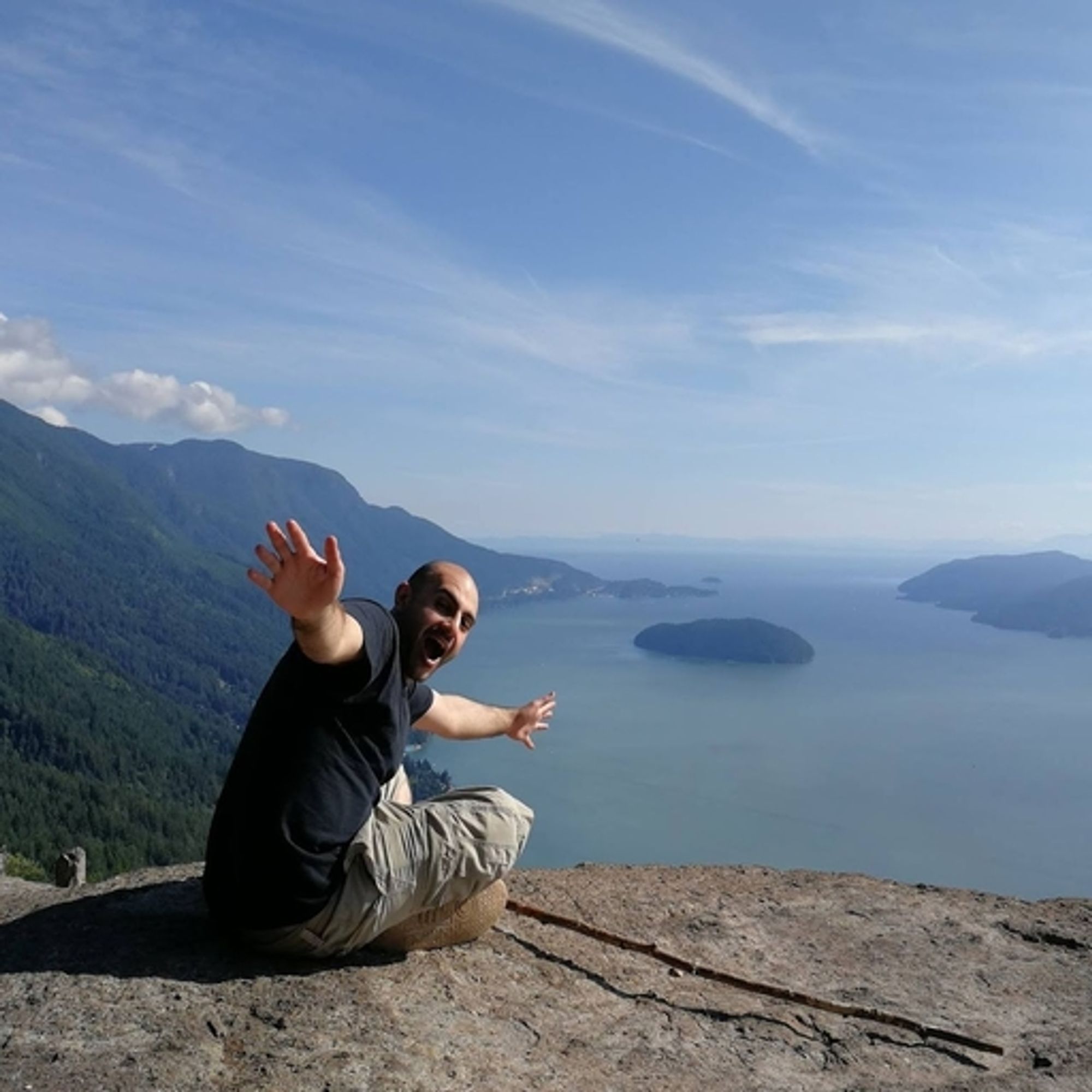
~ Ibrahim Elchami | Co-Lead
IoT/AI cloud architect | Postdoc, University of British Columbia, Canada
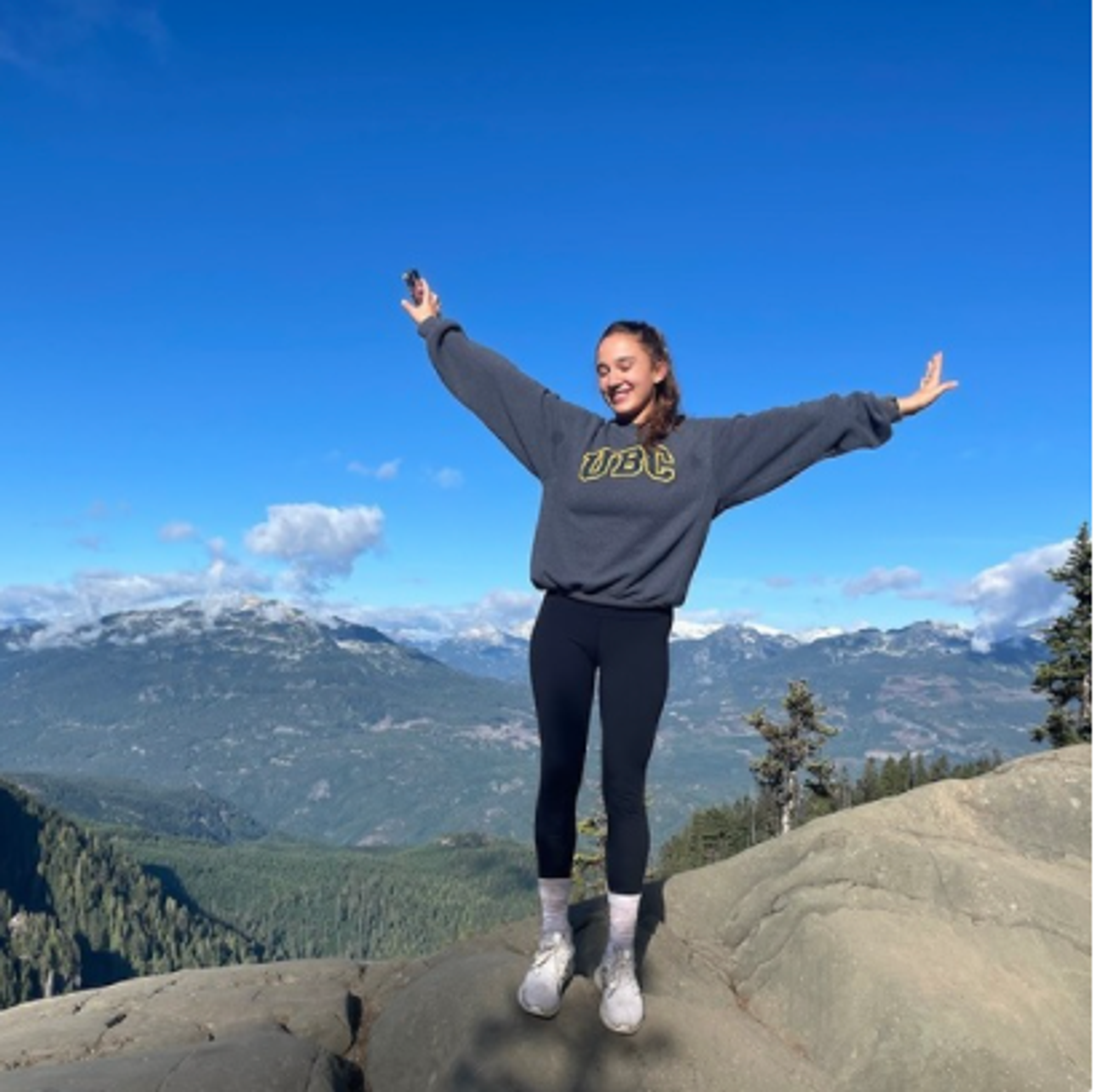
~ Isabel Todorova | Co-Lead
Drone expert | UBC Graduate @ University of British Columbia, Canada
❗ Meeting Link: Zoom
❗ Meeting Time: Mondays 6-6:30 PM ET on Mondays (starting Monday 23rd of May)
❗ Slack channel [Communication point]: Join by clicking here
❗ Dataset: Drone Images from 3 Parks
❗ Session Slides: Slides [including optional additional information]
❗ Session Videos: Session Videos
Project Overview
- Learn how to access, download and align remote sense imagery from public parks
- Practice organizing and structuring big datasets for streamlined use in custom/computer vision ML projects
- Develop a custom vision ML model using open sources tools, e.g. Yolov4, Yolov5. Model is used to
- [Object detection]: Count trees in a given image
- [Classification]: Classify trees in a given image into groups, such as deciduous or conifer.
- Learn how to quickly demo a ML classifier on images using a webapp (e.g. Flask API).
- Examine model performance and robustness when tested against seen/unseen regions across Canada (and practice implementing hyperparameter optimization)
- Compare model performance against cloud-based tools, e.g. CustomVision.ai from Microsoft.
- Optimize the model (e.g. TFLite, Yolov4-tiny) to run on a smartphone app, simple webapp, and/or run as an edge-AI/embedded-AI component.
- Minimal Project Goal: Develop a ML classifier that can correctly identify and count multiple tree types across different Canadian landscapes
- Stretch Goal:
- Use open-source tools to build a simple, user-friendly webapp makes it user-friendly enough for City employees to process the data in much easier ways.
- Write up a short article that examines this problem and share your findings
Tentative Project Timeline
# | Major Milestones | Expected time to finish |
1 | Get familiar with the project domain | 1-2 weeks |
2 | Download & process drone data | 1 week |
3 | Develop ML classifiers | 2 weeks |
4 | Testing / model parameter optimization | 1 week |
5 | Analyze model predictor importances | 1 week |
6 | Build a simple, quick webapp | 1 week |
Why join?
Aggregate Intellect hosts one of the most diverse ML communities in the world. Over the course of the working group
- Drones are super cool 😎 😎 😎 😎 😎 !!
- You’ll get an immersion into that community & walk out with some cool new friends.
- Advance your ML skills in remote sensing, and how to integrate multi-source data with different uncertainties in Python.
- There are $2500 in gifts budgeted for participants based on contribution. (A nice little push to encourage 😉)
- Contribute to a research study area to better understand the social-ecological interactions in urban environments, which in turn further contributes to building better tools for environmental justice, human health, well-being, and climate change
Looking forward to meeting everyone in our study group! Please feel free to reach out if you have any questions about the planned project.
This effort is being sponsored by our friends at
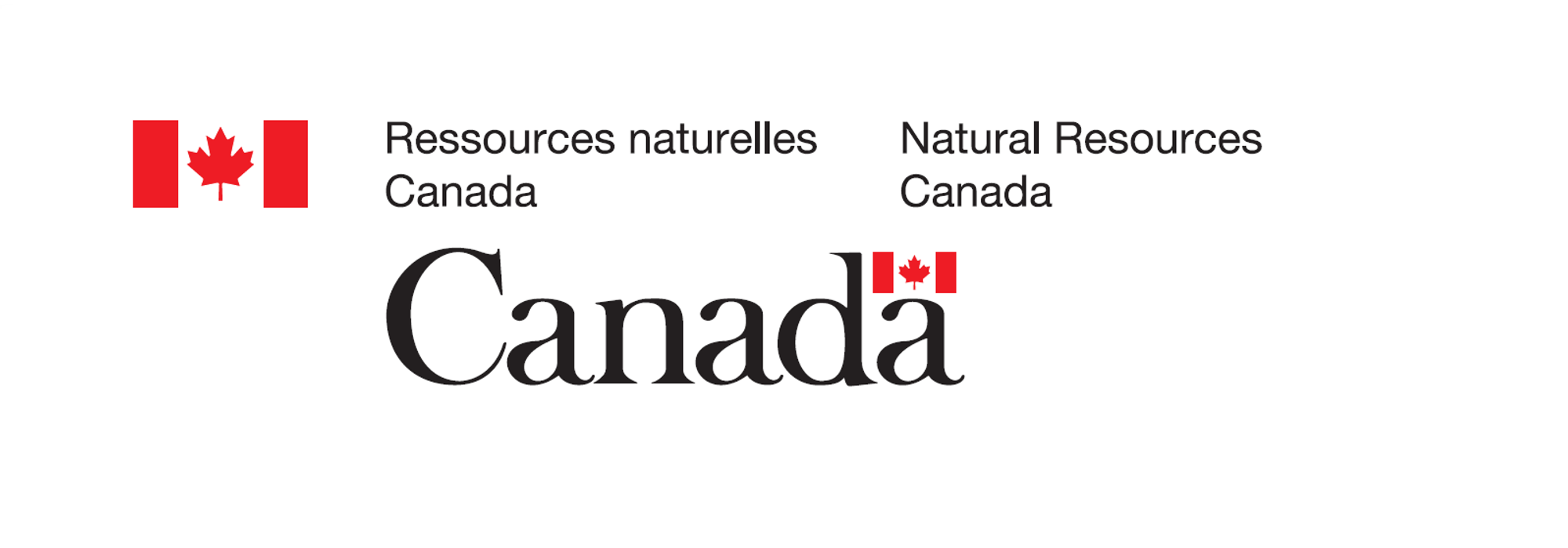